The recent 12th Annual Tribal Casino Executive Exchange in Carlsbad, California, US, was an enormous success, bringing together tribal gaming leaders to explore the impressive collection of emerging technologies that are already transforming casino operations.
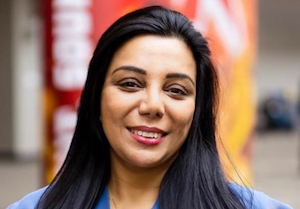
While the conference considered the benefits of facial recognition, innovations in responsible gaming and cutting-edge digital engagement strategies, one technology emerged as a game-changer for casino marketing: Causal AI, a form of artificial intelligence designed to identify and understand the cause and effect of relationships across data.
Traditional casino analytics have long relied on predictive models that identify correlations or patterns and relationships in player behaviour. These systems excel at answering standard “who-what-when-where” questions, such as who is visiting the casino, what games are they playing (and when), which promotions are they redeeming and what other services are they patronising? Traditional analytics do a wonderful job of identifying correlations that are often impossible to spot otherwise.
However, as I explained during my conference presentation, correlation doesn't equal causation – a distinction that is costing casinos millions in wasted marketing spend. Traditional casino analytics can tell you a lot about relationships in player data, but what they can’t tell you is the nature of those relationships and why they exist.
Consider this analogy I shared with attendees: both ice cream sales and shark attacks increase during the summer months. They're perfectly correlated, but it would be absurd to suggest that ice cream consumption causes shark attacks or vice-versa. A third factor – hot weather – drives both phenomena. Casino marketing is often susceptible to exactly this type of logical fallacy – that is, assuming a direct relationship between variables that doesn’t actually exist. For example, when a player receives free play and subsequently returns to the casino, traditional analytics assumes that the promotion caused the return. But what if the player had been planning to visit again anyway? How would traditional analytics know the difference? The answer is simple: it wouldn’t. It's the equivalent of giving a daily commuter a 25 per cent off gas coupon in the hope that they’ll visit your gas station, when in reality they are coming regardless of any incentives they might receive. They've already made the decision one way or the other because it's the only station on their way to work.
Enormous numbers of casino patrons behave in the same way. They’re happy to accept free play or other benefits, but they would have visited the casino regardless. It didn’t influence their behaviour; all that happened was that the casino wasted their money by inciting a guest that would visit regardless.
The real-world impact of marketing waste and how causal AI can help
This isn't merely theoretical. During the Native Nations Conference, numerous tribal casino operators shared familiar challenges: rising acquisition costs, declining marketing efficiency and diminishing returns on promotional spending. One operator confessed that they were still sending monthly mailers to players who had consistently visited every Tuesday for seven years without ever testing whether those incentives were actually necessary.
The financial implications of this sort of inertia are significant. Industry-wide, casinos distribute billions in comps and free play annually. Yet, causal AI implementations reveal that up to 25 per cent of this spend goes to players who would have gone anyway, representing hundreds of millions of dollars in potential savings had the casino known this wasn’t necessary. Unlike traditional predictive analytics, causal AI conducts non-stop virtual experiments across massive datasets to determine what actually causes player behavior. It doesn't just identify and map correlations; it identifies the specific factors that drive visitation, spending, and game preference.
Causal AI also integrates with existing casino management systems quickly and easily, requiring minimal implementation effort, which was a key point of interest for conference attendees concerned about IT complexity and staff training requirements. The point I was making to attendees was that if operators think it is challenging to implement and use AI, then they are not using an actual AI solution. True AI is intuitive and accessible, and, as such, Causal AI will integrate seamlessly with their existing workflows.
Beyond free play: unexpected insights
Conference discussions also revealed how causal AI's applications are extending far beyond optimising free-play distribution. Implementations across a wide range of properties have yielded some surprising discoveries. A central California casino learned that age, not just distance, determines what incentives drive visitation. Older players were willing to drive further for smaller rewards, while younger demographics required significantly higher incentives to do the same. A multi-property operator discovered that its cross-property marketing strategy was based on faulty assumptions. Contrary to conventional wisdom, established players – not new customers – were most likely to visit the operator’s sister properties.
One casino found that the physical distance between certain games and amenities strongly influenced both time spent gaming and revenue derived from food and beverage. A simple floor redesign based on these insights resulted in significantly higher revenues in both departments. Perhaps most surprisingly, causal AI showed that competition for players extended far beyond neighboring casinos as competitors aren't just other casinos. Thanks to causal AI, operators confirmed that they compete against every entertainment option out there including restaurants, sports bars, theatres and streaming services, each of which want their players' disposable income.
The path to implementation
The conference also showcased various approaches to AI adoption, from cautious experimentation to immediate full-scale implementation. Based on panel discussions and case studies presented, the most successful implementations appear to share several characteristics. Properties that established specific, measurable objectives saw faster ROI. Properties that started with a focused application before expanding – like optimising free play for a specific segment – tended to do better overall.
Casinos with app-based or text messaging capabilities were able to implement more personalised, timely incentives compared to those that relied entirely on monthly mailers. Operations that included casino hosts in the implementation process reported smoother adoption and better results overall.